引用本文: | 王飞,李正辉,李渝,等.基于数据序列分辨率压缩尺度优化的月度电量预测方法[J].电力系统保护与控制,2020,48(11):62-68.[点击复制] |
WANG Fei,LI Zhenghui,LI Yu,et al.Data series resolution compression scale optimization based monthly electricity consumption forecasting[J].Power System Protection and Control,2020,48(11):62-68[点击复制] |
|
本文已被:浏览 5411次 下载 1992次 |
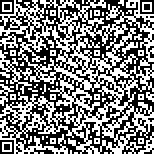 码上扫一扫! |
基于数据序列分辨率压缩尺度优化的月度电量预测方法 |
王飞,李正辉,李渝,王铁强,丘刚,郭怀东,马辉,王东升 |
|
(华北电力大学, 河北 保定 071003;国网新疆电力有限公司,新疆 乌鲁木齐 830018;国网河北省 电力有限公司,河北 石家庄 050021;北京金风慧能技术有限公司,北京 100176;新疆金风科技 股份有限公司,新疆 乌鲁木齐 830026;国网冀北电力有限公司,北京100054) |
|
摘要: |
准确的月度电量预测是指导电力部门安排运行计划和保障售电公司盈利能力的关键基础。针对基于人工智能算法电量预测模型在训练过程中存在样本分辨率选择不当导致预测精度降低的问题,提出了一种历史数据序列分辨率压缩尺度优化方法。首先将数据分辨率压缩尺度选择建模成一个优化问题。然后通过数据驱动方式进行求解。最后再利用长短期记忆(Long Short-term Memory, LSTM)神经网络实现月度电量预测,从而较好地实现了数据分辨率和电量预测步长之间的合理平衡以提升电量预测精度。利用美国PJM电力市场的历史电量数据对所提方法进行验证。仿真结果表明,较不进行分辨率压缩尺度选择所提方法具有更高的预测精度,同时LSTM网络在结合该方法时表现出了最佳的预测性能。 |
关键词: 电量预测 分辨率 尺度压缩 多步预测 长短期记忆神经网络 |
DOI:10.19783/j.cnki.pspc.200539 |
投稿时间:2019-05-15 |
基金项目:国家重点研发计划项目政府间国际科技创新合作重点专项(2018YFE0122200);河北省重大科技成果转化专项(19012112Z);国家电网公司科技项目(SGHE0000KXJS1800163) |
|
Data series resolution compression scale optimization based monthly electricity consumption forecasting |
WANG Fei,LI Zhenghui,LI Yu,WANG Tieqiang,QIU Gang,GUO Huaidong,MA Hui,WANG Dongsheng |
(North China Electric Power University, Baoding 071003, China;State Grid Xinjiang Electric Power Co., Ltd., Urumqi 830018, China;State Grid Hebei Electric Power Co., Ltd., Shijiazhuang 050021, China;Beijing Goldwind Smart Energy Technology Co., Ltd., Beijing 100176, China;Xinjiang Goldwind Science Technology Co., Ltd., Urumqi 830026, China;State Grid Jibei Electric Power Co., Ltd, Beijing 100054, China) |
Abstract: |
Accurate monthly electricity forecasting is the crucial basis to guide the operation plans arrangement of power grid corporation and guarantee the profitability of electricity retailers. To address the problem that improper selection of sample resolution during model training process based on artificial intelligence algorithms will seriously reduce the forecasting accuracy, a method for optimizing the compression scale of historical electricity data series resolution is proposed in this paper. First, the selection of data resolution compression scale is modeled as an optimization problem. Then, the data-driven method is used to solve the optimization problem. Finally, Long Short-Term Memory (LSTM) neural network algorithm is utilized to forecast the monthly electricity. As a result, a reasonable balance between data resolution and electricity forecasting step is achieved. The historical electricity data from PJM are utilized to verify the proposed method. The results show that the proposed method performs better than that without resolution compression scale selection, and the LSTM neural network combined with the proposed method shows the best forecasting performance. This work is supported by National Key Research and Development Program of China (No. 2018YFE0122200), Major Science and Technology Achievements Conversion Project of Hebei Province (No. 19012112Z), and Science and Technology Project of State Grid Corporation of China (No. SGHE0000KXJS1800163). |
Key words: electricity forecasting resolution scale compression multi-step forecasting long short-term memory neural network |