引用本文: | 刘谋海,胡军华,丁国栋,等.基于深度学习的电力监控视频超分辨率重建[J].电力系统保护与控制,2021,49(11):181-187.[点击复制] |
LIU Mouhai,HU Junhua,DING Guodong,et al.Super-resolution reconstruction of a power monitoring video based on deep learning[J].Power System Protection and Control,2021,49(11):181-187[点击复制] |
|
本文已被:浏览 5444次 下载 1541次 |
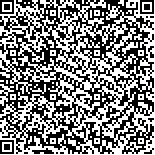 码上扫一扫! |
基于深度学习的电力监控视频超分辨率重建 |
刘谋海1,3,胡军华1,3,丁国栋2,黄瑞1,3,周纲1,3,余敏琪1,3 |
|
(1.国网湖南省电力有限公司,湖南 长沙 410004;2.长安大学信息工程学院,陕西 西安 710064;
3.智能电气量测与应用技术湖南省重点实验室,湖南 长沙 410004) |
|
摘要: |
针对电力监控视频有时分辨率不高问题,提出了一种基于深度学习的电力监控视频超分辨率重建方法。该方法首先采用卷积神经网络对输入视频帧进行特征提取,从输入视频帧序列两端按时间顺序将所有支持帧逐帧对齐到目标帧,并采用时间与空间自注意力机制将支持帧特征逐帧融合到目标帧。然后引入多尺度特征学习机制,对特征融合后的目标帧使用多分支特征选择重建网络进行超分辨率重建。最后采用公共数据集和电力视频数据集对该方法进行了训练。测试结果表明,所提方法的峰值信噪比与结构相似性指标均值分别达27.08和0.819,能有效提高电力监控视频的分辨率和视觉效果。 |
关键词: 电力系统 监控视频 超分辨率重建 深度学习 残差神经网络 |
DOI:DOI: 10.19783/j.cnki.pspc.200977 |
投稿时间:2020-08-12修订日期:2020-10-30 |
基金项目:国家电网科技项目资助(5216AB180006) |
|
Super-resolution reconstruction of a power monitoring video based on deep learning |
LIU Mouhai1,3,HU Junhua1,3,DING Guodong2,HUANG Rui1,3,ZHOU Gang1,3,YU Minqi1,3 |
(1. State Grid Hunan Electric Power Limited Company, Changsha 410004, China; 2. School of Information Engineering,
Chang’an University, Xi’an 710064, China; 3. Hunan Province Key Laboratory of Intelligent Electrical
Measurement and Application Technology, Changsha 410004, China) |
Abstract: |
The resolution of a power monitoring video is sometimes problematically low. Thus a super-resolution reconstruction model of such a video based on deep learning is proposed. First, a convolutional neural network is used to extract features of the input video frames. All support frames are aligned to the target frame from the two ends of the input video sequence in chronological order, and a time and space self-attention mechanism is used to fuse the support frame features to the target frame by frame. Secondly, a multi-scale feature learning mechanism is introduced, and a multi-branch feature selection reconstruction network is constructed to perform super-resolution reconstruction of the target frame after feature fusion. Finally, the model is trained with public datasets and power video datasets. The results indicate that the proposed method can effectively improve the resolution and visual effects of a power monitoring video, whose average value of the peak signal-to-noise ratio and structural similarity index reaches 27.08 and 0.819 respectively. |
Key words: power system monitoring video super-resolution reconstruction deep learning residual neural network |