引用本文: | 陈 峰,余 轶,徐敬友,等.基于Bayes-LSTM网络的风电出力预测方法[J].电力系统保护与控制,2023,51(6):170-178.[点击复制] |
CHEN Feng,YU Yi,XU Jingyou,et al.Prediction method of wind power output based on a Bayes-LSTM network[J].Power System Protection and Control,2023,51(6):170-178[点击复制] |
|
本文已被:浏览 6222次 下载 1193次 |
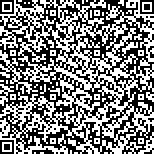 码上扫一扫! |
基于Bayes-LSTM网络的风电出力预测方法 |
陈峰1,余轶1,徐敬友1,杨洁1,陈可1,张天东2,郭露方3,郑子健3,胡钋3 |
|
(1.国网湖北省电力有限公司经济技术研究院,湖北 武汉 430077;2.国网石家庄供电公司,
河北 石家庄 050000;3.武汉大学电气与自动化学院,湖北 武汉 430072) |
|
摘要: |
为提高风电出力的预测精度,提出一种基于Bayes优化的长短期记忆人工神经网络(long-short term memory, LSTM)的预测模型。首先,利用经验模态分解对风电历史出力序列进行分解,并对各分量及原始数据分别提取8个统计特征量,与预测前6个时刻出力值共同组成预测特征集。然后,采用绳索算法(least absolute shrinkage and selection operator, LASSO)从预测特征集中提取具有统计意义的特征子集,作为预测模型的输入。最后,提出基于Bayes超参数寻优的LSTM网络优化方法,以提高预测精度。选取湖北某市风电出力历史数据进行预测实验,结果表明:相较于BP神经网络、SVM、RBF网络、GRNN网络等预测模型,所提模型预测精度较高,特征提取方法较为合理。 |
关键词: 风电出力预测 深度学习 Bayes优化 特征提取 |
DOI:10.19783/j.cnki.pspc.220817 |
投稿时间:2022-05-31修订日期:2022-09-21 |
基金项目:国家自然科学基金面上项目资助(51977160);国网湖北省电力公司科技项目资助(SGHBJYOOPSJS2200130) |
|
Prediction method of wind power output based on a Bayes-LSTM network |
CHEN Feng1,YU Yi1,XU Jingyou1,YANG Jie1,CHEN Ke1,ZHANG Tiandong2,GUO Lufang3,ZHENG Zijian3,HU Po3 |
(1. State Grid Hubei Electric Power Company Limited Economic Research Institute, Wuhan 430077, China;
2. State Grid Shijiazhuang Power Supply Company, Shijiazhuang 050000, China; 3. School of Electrical
Engineering and Automation, Wuhan University, Wuhan 430072, Chin) |
Abstract: |
To improve the prediction accuracy of wind power output, a model using a long- and short-term memory (LSTM) artificial neural network based on Bayesian optimization is proposed. First, empirical mode decomposition is used to decompose an historical output series of wind power, and eight statistical features are extracted from each component and the original data respectively. In this way a prediction feature set is formed together with the output values at the first six moments of prediction. Then, a least absolute shrinkage and selection operator (LASSO) algorithm is used to extract the feature subset with statistical significance from the prediction feature set as the input to the prediction model. Finally, an optimization method of an LSTM network based on Bayesian super-parameters optimization is proposed to improve prediction accuracy. The historical data of wind power output in a city in Hubei Province are selected for a prediction experiment. The results show that, compared with a BP neural network, support vector machine (SVM), radial basis function (RBF) network, general regression neural networks (GRNN) network and other prediction models, the proposed model has higher accuracy and the feature extraction method is more reasonable. |
Key words: wind power output prediction deep learning Bayes optimization feature extraction |