引用本文: | 苏向敬,朱敏轩,宇海波,等.基于频谱注意力和无交叉联合分位数回归的海上风电功率超短期概率预测[J].电力系统保护与控制,2024,52(21):103-116.[点击复制] |
SU Xiangjing,ZHU Minxuan,YU Haibo,et al.Ultra-short-term probabilistic forecasting of offshore wind power based on spectral attentionand non-crossing joint quantile regression[J].Power System Protection and Control,2024,52(21):103-116[点击复制] |
|
本文已被:浏览 1155次 下载 497次 |
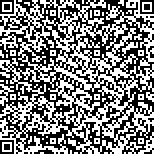 码上扫一扫! |
基于频谱注意力和无交叉联合分位数回归的海上风电功率超短期概率预测 |
苏向敬1,2,朱敏轩1,3,宇海波4,李超杰5,符杨1,2,米阳3 |
|
(1.教育部海上风电技术工程研究中心(上海电力大学),上海 200090;2.上海电力大学海上风电研究院,
上海 200090;3.上海电力大学电气工程学院,上海 200090;4.国网四川省电力公司甘孜供电公司,
四川 甘孜 626000;5.新南威尔士大学电气工程与通信学院,悉尼2052,澳大利亚) |
|
摘要: |
海上风电功率波动性和随机性强,其时序数据既存在时间上的全局性关联,又包含大量局部的短期噪声;同时,现有概率预测模型普遍采用的分位数回归方法存在分位数交叉问题,严重影响了预测结果的精度与合理性。针对上述挑战,提出了一种基于频谱注意力和无交叉联合分位数回归的海上风电功率超短期概率预测方法。首先,结合长短期记忆网络(long short-term memory, LSTM)模型和频谱注意力模型,挖掘风电功率时序数据的全局长期依赖,并滤除局部噪声。其次,在此基础上构建了一种基于联合分位数回归的损失函数模型,通过共享各分位数预测任务间的信息,自主动态调节各分位数损失的权重,避免分位数交叉问题。最后通过真实算例分析表明,相比于现有概率预测模型,所提模型的锐度指标提升了6%以上,连续等级概率分数提高了10%以上,故具有更高的预测精度,并有效解决了分位数交叉问题。 |
关键词: 海上风电 概率预测 频谱注意力机制 分位数回归 |
DOI:10.19783/j.cnki.pspc.240173 |
投稿时间:2024-02-10修订日期:2024-05-07 |
基金项目:上海市教育委员会科研创新计划项目资助(2021-01-07-00-07-E00122) |
|
Ultra-short-term probabilistic forecasting of offshore wind power based on spectral attentionand non-crossing joint quantile regression |
SU Xiangjing1,2,ZHU Minxuan1,3,YU Haibo4,LI Chaojie5,FU Yang1,2,MI Yang3 |
(1. Engineering Research Center of Offshore Wind Technology Ministry of Education (Shanghai University of Electric
Power), Shanghai 200090, China; 2. Offshore Wind Power Research Institute, Shanghai University of Electric
Power, Shanghai 200090, China; 3. College of Electrical Engineering, Shanghai University of Electric Power,
Shanghai 200090, China; 4. State Grid Sichuan Ganzi Electric Power Supply Company, Ganzi 626000, China;
5. School of Electrical Engineering & Telecommunications, University of New South Wales, Sydney 2052, Australia) |
Abstract: |
Because of the high fluctuation and random nature of offshore wind power, time-series data contains not only global correlations but also a large amount of short-term local noise. In addition, existing probabilistic forecasting studies are commonly based on quantile regression methods which suffer from the quantile crossing problem and unreliable forecasting results. Therefore, this paper proposes an ultra-short-term offshore wind power probabilistic forecasting method based on a spectral attention and a crossing free joint quantile regression. First, combined with the long short-term memory (LSTM) model and spectral attention model, the global long-term dependence is mined and local noises are filtered in the time-series data. Then, a joint quantile regression loss function model is built to avoid the quantile crossing problem by dynamically adjusting the loss weights and sharing information among all the quantile regression tasks. Real case studies demonstrate that, compared to LSTM and TCN, the proposed model has improved the sharpness of quantile forecasting by over 6% and the continuously ranking probability score by over 10%, with superior prediction accuracy and the quantile crossing problem well addressed. |
Key words: offshore wind power probabilistic prediction spectral attention mechanism quantile regression |